Major Depression Disorder detection on EEG-Based with the Convolution Neural Networks approach improved with the Inter-trial phase clustering
Rathanon Suwanteerangkul and Yuttana Kitjaidure*
Department of Electronics Engineering, Faculty of Engineering, King Mongkut's Institute of Technology Ladkrabang, Thailand
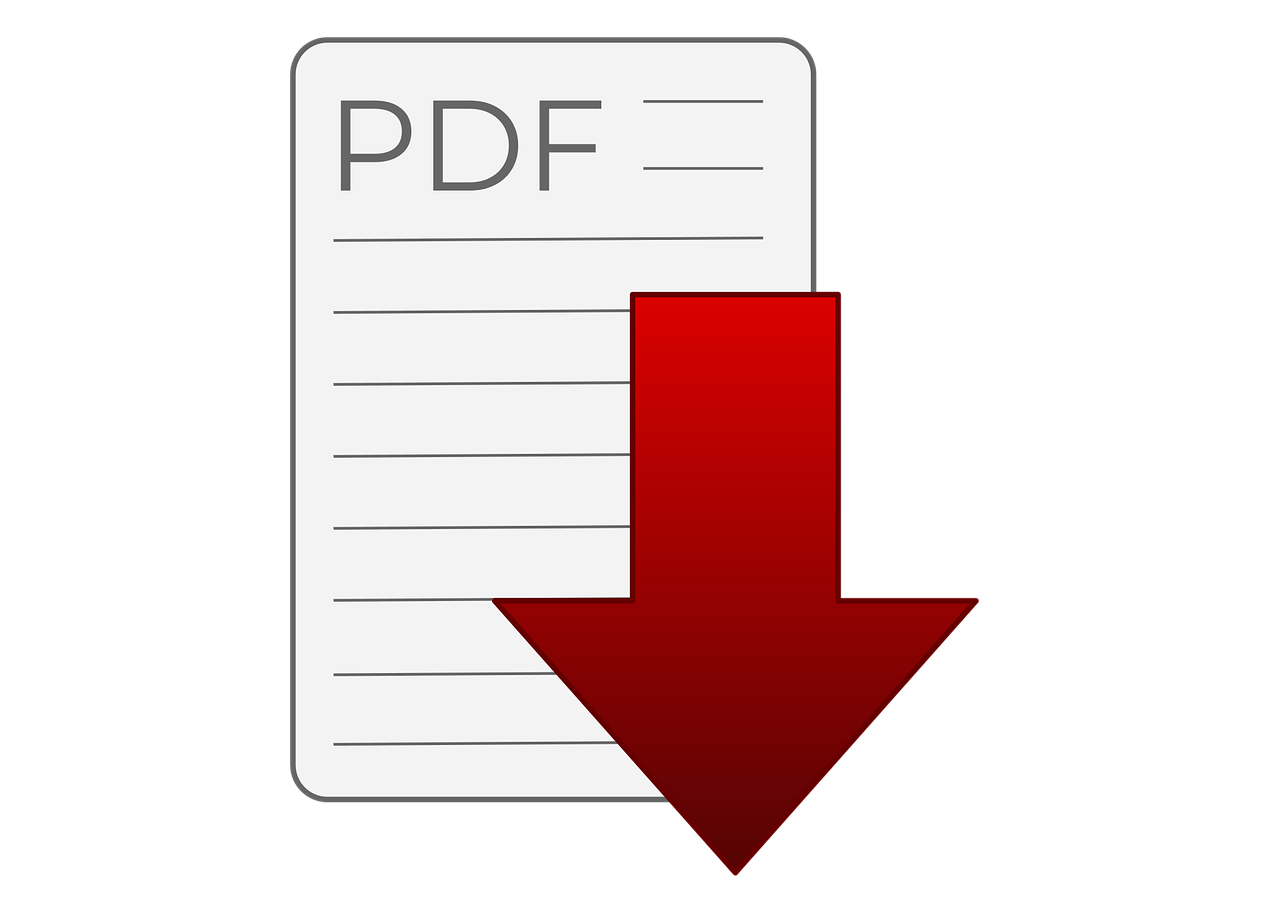
Abstract
Nowadays, Major Depression Disorder is still a concern about human mental health, and there are significant challenges in the diagnosis. There are still limitations in the traditional method used to classify patients from healthy groups and required specialized personnel such as a psychiatrist or psychologist. The aim of this study is to introduce the ability of a deep learning approach to detect abnormalities in EEG (Electroencephalogram) signals of patients with depression. The EEG features are based on the Time-Frequency Analysis of EEG signals from the Morlet wavelet transformation, also known as ERPs (Event-Related Potentials) and ITPC (Inter-trial phase clustering), which is complex and challenging to assess by humans. We proposed a deep learning model that are integrating the two Convolution Neural Networks (CNNs) models to learn both EEG features at the same time to increase efficiency in classification. The result shows that the concatenation CNNs between two input's EEG features received accuracy in classification at 91.60% compared with general CNNs that use single input ERPs with accuracy only 83.33%. Also, we are comparing with a machine learning approach, such as KNN (K-nearest neighbor), that received an accuracy of 67.00%.
|